The annual New Year’s Reception held by Egon Zehnder’s Munich office brings together senior business leaders to engage on big ideas and expand their vision beyond their own professions. The 2018 reception, the 25th in the series, shone the spotlight on the exponential advance of technologies such as artificial intelligence (AI), nanotechnologies, and digital biology – all of which promise huge opportunities and dramatic disruption across business and society.
The keynote speaker was Neil Jacobstein, Chair of AI and Robotics at Singularity University at NASA Research Park, and Distinguished Visiting Scholar at MediaX at Stanford University. A deeply interdisciplinary thinker, Mr. Jacobstein has a keen sense of how the arts and the sciences can integrate. At our reception, he shared vivid insights on how businesses can adapt to and succeed in “exponential futures” – and reflected on the trade-offs that both the private and public sectors must make if they are to strike a “technology-human balance”. He was joined on stage by Christoph Keese, the economist and author of the award-winning book Digital Germany. In this publication we share both an edited version of Mr. Jacobstein’s address, and excerpts of the dialogue between him and Mr. Keese.
Leo Barth, Managing Partner of Egon Zehnder in Munich, opened the reception with a reflection on the challenges that exponential advances in technology pose to leaders today. “We’re all accustomed to the fact that everything is constantly changing,” he said. “Agility and creativity are essential to leading a company successfully today.” The problem, Mr. Barth observed, is that “exponential developments, unlike linear ones, are very hard to anticipate for the human brain.”
By way of analogy, Mr. Barth recounted the famous tale of the Indian king who wanted to reward Sita, the inventor of chess: “The king was at first offended by Sita’s irritatingly modest request, just one grain of rice on the first square of his chess board, and then double the amount on each of the following squares, 2 grains, 4, 8, 16, and so on.” By square 64, however, the king owed Sita 277 billion tons of rice – more than 400 years of global rice production.
As Mr. Barth emphasized: “Our future technologies might advance at this kind of speed, creating ever-greater and furtherreaching opportunities. Everybody is trying to be the fastest to turn these opportunities into successful business models. In these times, though, our responsibility as leaders goes much further. It is no longer enough just to position your company strongly for the digital era; we’re also responsible for achieving the right balance in this era. What will tomorrow’s working world look like? How can we achieve social cohesion? How can we make sure that as many people as possible benefit from technological advancements?”
Those were questions that prompted both powerful perspectives from Mr. Jacobstein and Mr. Keese, and rich dialogue between our guests. We hope this publication inspires ideas and innovation both from those present at the reception, and from a much wider audience of leaders.
What will it take to adapt successfully to an accelerating technological future? Corporations, governments, and individuals are hungry for answers to this question. However, there is no one technological future for which organizations or individuals can prepare. There are multiple possible futures, with a varying mix of technologies and features, each accompanied by uncertainty in assigning a probability to its actual occurrence. The good news is that some features of the most probable technological futures can be forecast with reasonable certainty. Further, the actual future is not yet written – it is still in play, and we are all co-authors. How do we improve our likely outcomes in the future? The best way is to honor uncertainty by taking actions that are smart bets in most of the futures, informed by our understanding of the most probable course of events. In other words, invest in the actions that have the most survival and success value in the set of futures you and your organization are likely to face. This may seem obvious, but it is not. Some of the most probable futures ahead will be shaped by exponential technologies. These technologies are moving much faster than most people realize, and their advance is accelerating.
Exponential technologies are not intuitive. They do not reward linear thinking (1, 2, 3, 4, 5, 6 ...). Every 18 to 24 months they double in their delivered performance of speed, memory, or other parameters. Their performance improvement increases exponentially (2, 4, 8, 16, 32, 64, 128 ...). Examples are artificial intelligence (AI), robotics, synthetic biology, and digital manufacturing. These technologies start off underperforming against linear expectations, and then vastly exceed them. Synthetic biology is rapidly increasing our ability to read and edit DNA, with decreasing cost and increasing precision. Synthetic biology utilizes DNA as software for programming and editing biological systems. Digital manufacturing includes rapidly advancing 3D printing at the micro scale, and a range of nanotechnology.
Nanotechnology has a conventional definition of utilizing the chemical and physical properties of materials that change at the scale of a billionth of a meter. However, we are just beginning to see lab-scale demonstrations of integrated nanosystems, and the atomically precise manufacturing that they will enable. Atomically precise manufacturing is a future technology that will alter our relationship with molecules and matter – like the computer altered our relationship with bits and information. Specifically, it will eventually enable inexpensive and ubiquitous 3D programming of both small and very large systems.
The key to remember is that utilizing exponential technologies effectively requires exponential thinking that matches the pace of these technologies – and their potential to generate wealth and trans m the world. There is a massive economic premium on paying attention to these trends. Business-as-usual models that worked in the past need to be upgraded to track and systematically harness the opportunities of exponential technologies.
What does it actually mean to think exponentially?
Here are five steps.
1. You understand the difference between technologies that have linear improvement curves and those that are exponential.
2. You have multiple examples of how fast and effectively exponential technologies are improving.
3. You have collected case studies of how exponential technical improvements have translated to practical business and organizational advantages in many areas.
4. You know the practical problem-solving and business power that could be gained by your specific business harnessing exponential technologies like AI and robotics, and you understand that if you don’t use them, your competition will.
5. You have organized yourself and configured your organization to utilize exponential technologies systematically and responsibly in every aspect of your business – including transforming your business model if it is becoming obsolete.
We can illustrate steps 2 and 3 with examples from AI.
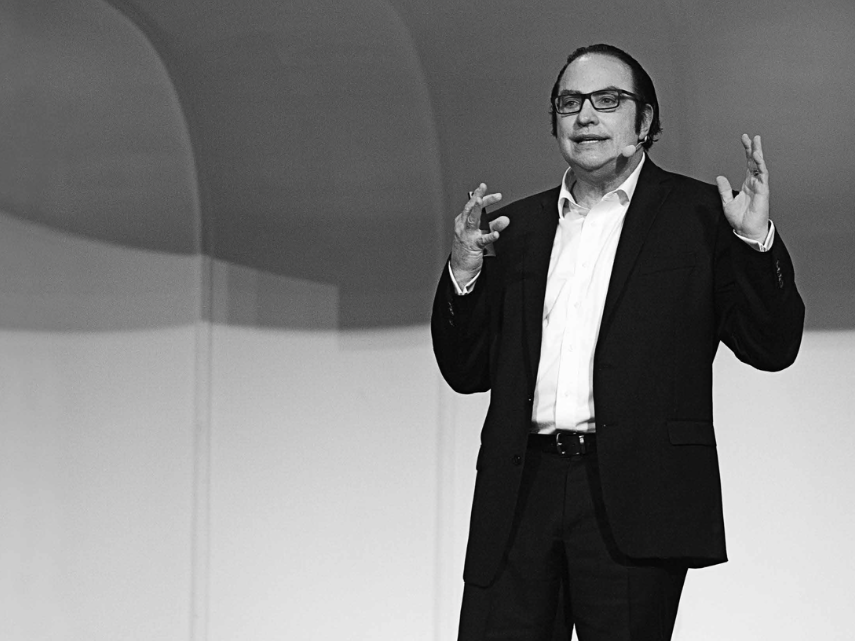
AI is Improving Rapidly
When Amazon introduced the AI-based Alexa agent in 2014 on their Echo-connected speaker, Alexa had 15 skills, or things you could verbally ask it to do. These skills were very basic, such as providing the time, streaming music, setting a timer or calendar date, providing news or sports scores, and ordering items from Amazon. In August of 2015, Alexa had three skills developed by vendors independent of Amazon. By September of 2017, Alexa had over 20,000 independent vendor-developed skills that could be performed in response to verbal requests. That is faster-than-exponential growth.
When comparing the complexity of chess to the Chinese strategy game Go, chess has about 35 alternative moves per turn, and Go has up to 250 alternative moves per turn. Thinking many moves ahead in Go is extremely challenging. In October of 2015 Alphabet’s DeepMind team played its AlphaGo against Fan Hui, then the European Champion in Go. AlphaGo won five games out of five. In March of 2016, AlphaGo beat Lee Sedol, the Korean World Champion in Go, four games to one. This win shocked many members of both the Go and AI communities. In May of 2017, AlphaGo Master beat Ke Jie, the Chinese number-one ranked World Champion in Go, three games to zero. Up to this point, AlphaGo was learning Go from thousands of Go games played by human Go players. However, in October 2017, DeepMind announced AlphaGo Zero that learned Go from scratch, playing against itself, starting with only the rules of Go. AlphaGo Zero surpassed the strength of AlphaGo Lee in three days by winning 100 games to zero, reached the level of AlphaGo Master in 21 days, and exceeded all the previous Go players in 40 days. AlphaGo Zero is as far ahead in Go skill level of the European Champion Fan Hui as Fan is ahead of a serious amateur Go player. The reason why this matters is that AIs have now outperformed human world champions in one of the most complex strategy games. This points unambiguously to the accelerating current and future business potential of AI.
Three Practical AI Case Studies
There are thousands of potential case studies, but these three will provide a starting point. For more examples of innovative applications of AI, explore the Innovative Applications of AI conference papers.
Case 1.
AI in Insurance Services. Lemonade.com is a highly automated insurance company that is now using AI and machine learning in every aspect of the business, from sales, to insurance risk analysis, to claims adjustment, to customer service. It has also transformed the traditional business model, taking 20 percent of premiums as a fee, and allowing clients to donate a portion of their unclaimed premiums to charity.
Case 2.
AI in Energy Efficiency. Jim Gao was an Alphabet data center manager
in 2014 who invested his 15 percent free time in improving power utilization efficiency in the data center. Using machine learning and help from the DeepMind team, Gao was able to improve the energy efficiency of the data center by a whopping 40 percent. Note that improvements in energy efficiency often have the highest return on investment.
Case 3.
AI in Medicine. An algorithm developed by Stanford computer scientists in 2017 can sift through hours of heart-rhythm data generated by wearable monitors to find sometimes life-threatening irregular heartbeats, called arrhythmias. The algorithm performs better than trained cardiologists on 14 types of arrhythmias, and can sort through data from remote locations where people don’t have routine access to cardiologists. There are similar cases of AIs outperforming radiologists at diagnosing pneumonias and ophthalmologists at diagnosing diabetic retinopathies.
Harnessing Exponential Technologies
Based on these three cases, it is easy to see that AI and machine learning are now able to provide task performance at human level and above in some complex arenas. While AI technology can’t currently replace an empathetic psychologist or massage therapist, it is able to provide cost-effective problem-solving power in a wide variety of practical applications that do not require “common sense” or general intelligence. The business applications are limited more by traditional business models and human imagination than by limitations in machine learning. The adoption rate of AI is now racing ahead, and those businesses that don’t get it, or don’t act swiftly and responsibly, will likely suffer the consequences of extremely empowered new competition.
Synthetic biology is also racing ahead. It used to cost a million dollars to do human DNA sequencing, and the cost now is approximately 100 dollars and headed downward. Helix is an app store for DNA data and optimizing your performance. It is now possible to identify disease subtypes as in diabetes, and characterize the microbiome and its multiple roles in health and disease. The new CRISPR/Cas9 technology enables increasingly precise editing of human DNA, including eliminating single base pair diseases, and in the years ahead potentially adding new augmentations associated with the best performing genes for specific traits.
Building nanosystems is now a research frontier for integrating individual nanoscale devices. Much of the current work on small systems applications focuses on microsystems that are three orders of magnitude larger than the nanoscale. For example, a team at Cornell has made a micro-scale robot exoskeleton that can rapidly change its shape upon sensing chemical or thermal changes in its environment. These machines could become a powerful platform for robotics at the size scale of biological microorganisms. These systems could converge with AI, by incorporating computational power in an object the size of a cell.
Business Transformation and Social Response
Given the speed and depth of exponential technology-driven competitive landscape transformations, businesses will need to take some specific actions. First, they will have to forecast how exponential technologies will impact their specific business sectors. Second, they will have to increase their rate of experimentation with fundamentally new products, services, and business models. But if they attempt to do this inside old business structures, an antigen-antibody reaction is almost guaranteed. So these experiments will need to take place outside of the organization, or on its edge. Third, risk-taking must be rewarded and experimental failures accepted without negative consequence to employees. Finally, every aspect of the business must be reframed in light of what is possible now with new technologies – even if it cannibalizes “cash cow” parts of the business.
Addressing Risks and Other Social Concerns
While there is no question that cost-effective AI and robotics applications will provide some new employment, it is the ratio of new jobs to jobs displaced that matters with respect to social concerns about under- and unemployment. These concerns will have to be addressed either by proactive access to retraining education and various basic income schemes, or by managing the consequences of social unrest. It is possible, but unlikely, that under- and unemployment will not become a short-term problem in a long-term future of widespread technological abundance. Adapting effectively to the likely scenarios, even with inherent uncertainty, would suggest covering the downside risks with greatly improved access to high-quality education and a variety of basic income schemes and social services, including counseling in managing self-esteem over the human life cycle.
In addition, there is concern that in the decades ahead, AIs could exceed human intelligence – and that, even without malevolence, their values and goals could drift from those beneficial to humans. A proactive approach to the potential risks of superintelligent AIs is already driving research and development on improved software specification, validation, security, and new control strategies.
The benefits of synthetic biology for human health and flourishing are many and increasing, but the technology also enables many possi- bilities that challenge human values. For example, in addition to curing deadly diseases, CRISPR/Cas9-based editing tools could enable a variety of private labs and governments to super-enhance intellectual or physical abilities. In some scenarios, the thoughtful use of this technology could lead to human flourishing; other uses could lead to super-soldiers or a split-off of the human species. Since the 1970s, however, molecular biologists have taken the long view and generated ethical guidelines for the use of new and emerging biotechnologies. Social dialogues around these ethical guidelines will become more complex as the technologies mature and the business applications expand dramatically.
Nanosystems are in embryonic states of development, and they could lead to unprecedented wealth. Wealth distribution will remain a challenge, but it will become progressively easier to distribute the benefits of these technologies as they increase in performance and decrease in price. Consider the rapid global spread of cellphone technology. However, for decades researches have recognized the potential future risk of nanosystems potentially replicating out of control. While it is clear that nanosystems can be built specifically with multiple and redundant safety systems that can avoid this problem, rogue actors could build systems that bypass the safety guidelines. The best defense against this potential future scenario is twofold: technical and ethical education on the best practices of nanosystems design, and continuous research and development on powerful immune systems to rapidly detect and shut down malevolent actions from AIs, biotechnology, or nanosystems.
The control strategies associated with each of these technologies could also contribute to many significant upside scenarios for society. We have the opportunity to use increasingly capable exponential technologies to address humanity’s “grand challenge” problems – including energy, the environment, global health, security and conflict resolution, education, water, food, disaster response, and prosperity distribution. There is a variety of educational programs available globally from Singularity University and other institutions that explore the benefits, risks, and many possibilities for human flourishing with exponential technologies.
Christoph Keese: We all know that Artificial Intelligence (AI) has been around for a very long time. Even in the 1960s and 1970s, scientists talked about the breakthrough of AI. It never happened. And it became exponential only three, four years ago with the advent of neural technology and deep learning. Could you give us your insight on why this is becoming exponential at this point in time?
Neil Jacobstein: Actually, my point of view is that it’s been exponential the whole time. When you start out with an exponential technology, you can barely see it happening. It’s almost imperceptible. And it takes a very long time, sometimes decades, before you see the results. So I think that the exponential trend in computing happened way back with relays and vacuum tubes. And the exponential trend with AI has been going on since AI began. In fact, rule-based systems were able to work in industry, manufacturing, banking, and biology. But they weren’t the best technology: they were hard to build and they were brittle. Now we have largely replaced rule-based systems with pattern-recognition systems based on neural networks, like deep learning. That has accelerated the advance in the field. But it’s always been exponential: we just couldn’t see it before.
Christoph Keese: There is a very successful AI company in Germany, called Arago. [Its founder] Chris Boos recently made a fascinating presentation arguing what the limits of AI will be, at least during our lifetimes. He says there are basically three different types of intelligence. The lowest type is simply pattern recognition, which humans do all day long, and which is in the process of being solved by computer intelligence. The second is reacting to pattern recognition – the machine comes up with the correct answer. This third, he says, is what we call empathy. And his argument is that none of us is going to see AI performing emotions or empathy that would pass the Turing test, where we could confuse humans and computers. But much of the public worry is about empathy – computers going out, hating us, and wanting to kill us. What is your viewpoint? Is there stuff that computers in our lifetimes will never be able to do?
“Are we going to see worldwide competition that is strong and fierce and vivid and energetic, or are we going to see a trend towards monopoly?”
Christoph Keese
Neil Jacobstein: On the second type, reacting to pattern recognition, we call that inference. And AIs are doing inference in a very credible way today. They don’t do inference the same way that humans do, but in some cases they exceed performance in inference.
As to empathy, we have systems that can convince humans that they’re being empathic, but I would claim they are not experiencing empathy. The machines are basically hijacking humans because they’ve been taught the rules of how to convince or fool a human into thinking that they’re empathetic. They’re mimicking the outward signs of empathy. There’s a guy named Paul Ekman, who has characterized 174 micro facial expressions. You can program those into a robot, and you can elicit the feeling on the part of humans: “Oh, this robot is being empathic with me.” But that’s not the same thing as the robot actually experiencing empathy. So I do think that, when it comes to passing a Turing test, you might be able to fool people into thinking that the AI is being empathic, but I think it’ll be a while before they actually experience empathy for themselves. I don’t think that’s a question of it not happening in our lifetimes for two reasons. One, I think that’s likely to happen in the next 15 to 25 years, could be sooner. And second, I think we’re going to live a very long time, longer than most people in this room expect.
Christoph Keese: What you’ve been saying has huge consequences for society and for business. [For example], when you’re recovering from surgery you need empathy, you need caring. So you could be much better off going to a nurse than to a doctor. So it could very well be that the doctor loses his job, while the nurse keeps hers. And that job is maybe even paid much better than the doctor because she cannot be sub- stituted by a machine. I would be interested in your opinion on that.
Neil Jacobstein: There was a book written in 1976 by Jerrold Maxmen, a psychiatrist at Yale, called The Post-Physician Era. And it argued something like that. But the scenario took much longer to unfold than he thought it would. My observation is that people don’t just go to doctors for a diagnosis. Real empathy with patients and helping patients understand the interpretation of the diagnosis is going to be very important. Because you don’t want a machine looking at your x-ray or your MRI and saying, “Oh, you’ve got cancer, 4.5 months to live.” That’s not okay for many reasons. You might have lots of alternative treatments that are available to you. You might need some time to think about it. There’s lots of things that go into that dialogue, and I don’t think our AIs are up to the task right now.
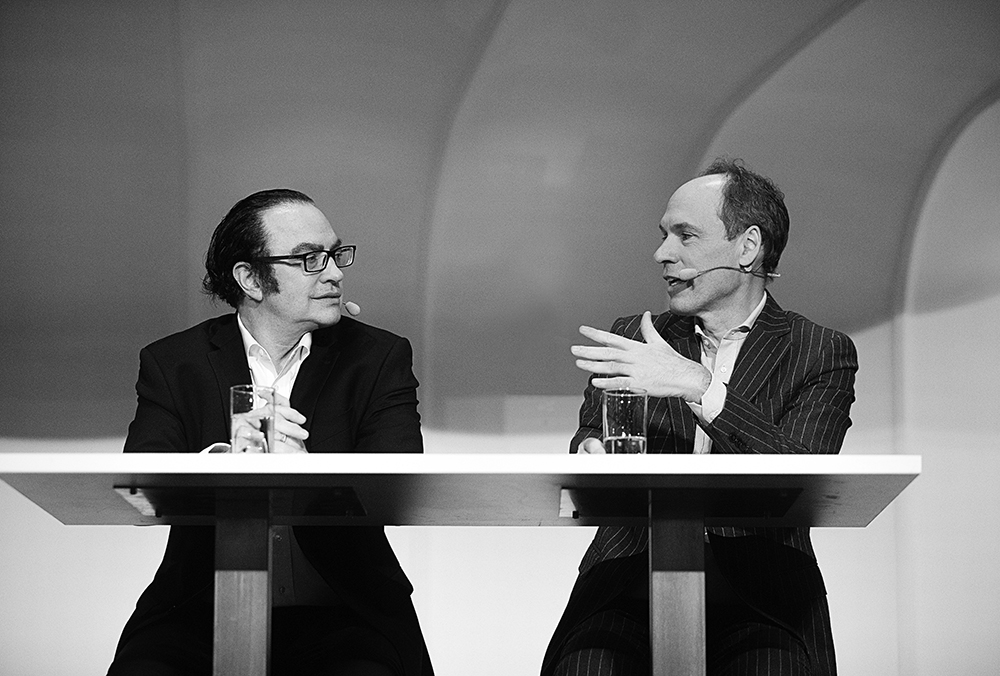
Christoph Keese: It’s interesting that so much of the AI capacity in the world – both intellectual capacity and company capacity – has been acquired by the big corporations like Amazon, Facebook, and Google. They acquired DeepMind. And when you look at it from a geopolitical viewpoint, much of the investments have been made in the United States and in China and very little in Europe, and Germany especially. So are we losing out, and even if we woke up today, is there enough left to buy, and talent to hire, after the big corporations have gone shopping over the past three to four years?
Neil Jacobstein: Although it’s true that a lot of great talent has been sucked up by big AI companies, the good news is that you can now crowdsource your talent from Kaggle, which allows companies to put up prize money and have 200 or 300 AI science teams compete for the solution to your problem. And of course, with that, you have to put out your data and your problem. If you don’t want to do that, you can go to experfy.com, which allows you to just put your problem out and not your data, if you’re worried about data privacy, and you can crowdsource your AI scientists that way and get very good results. (In the spirit of full disclosure, I’m an investor there.) The critical thing here is that you can crowdsource your talent. You don’t have to have deep bench strength inside your company. And I think that we’re going to find that many more people are going to study machine learning. We’re going to see that populations that have been disenfranchised have many brilliant people. In Africa, in Asia, we are seeing increased participation in AI, and the number of women in computer science is increasing. There are many geniuses there that are basically untapped for computer science. So I think there’s lots of talent that we haven’t recruited yet.
Christoph Keese: What are the most valuable data these days? What is it, data coming out of cars or medical data? If you were to put your hand up, if you had one wish and you could say, “Oh, this bulk of data should be mine,” what would you pick?
“There will be immense competition rather than monopoly. We’re going to have millions of AIs.”
Neil Jacobstein
Neil Jacobstein: I think that probably the area that has the highest potential for growth would be health data. But it would be a particular kind of health data, not just body temperature and heart rate and things like that, but deep data about people’s genomic sequence and the phenotypic expression of their genomic sequence. The correlation between their diet and exercise patterns and their patterns of health and disease really matters. I think that most of us feel like we can just kind of mosey along in our lives and do reasonably well with diet and exercise. And we figure that will get us most of the life that we have coming to us. But a different perspective would be that, if you really pay attention to diet and exercise and genomic sequence and monitoring your health, you might be able to squeeze a lot more life and useful life ... not a diminished life as you get older. That is an opportunity that is available to every person in this audience. And it’s going to take the intelligent management of your health data in order to do that.
Christoph Keese: When you look at the AI landscape in the future, Neil, are we going to see worldwide competition that is strong and fierce and vivid and energetic, or are we going to see a trend towards monopoly?
Neil Jacobstein: I think that there will be immense competition rather than monopoly. It’s easy to throw in the towel and say, “Oh, Google and Facebook and Amazon, they have all the marbles. And they’re just going to run away with things.” I don’t think that’s going to happen. I think there’s going to be immense competition from all over the world. And we won’t have one gigantic Skynet AI. We’re going to have millions of AIs. And they will permeate the fabric of everyday life so much that we won’t even call it AI anymore. We’ll just call it everyday life. We’ll just expect it to be there.