Executive leadership teams are all asking one big question: How should we be handling Artificial Intelligence?
AI is rapidly transforming the business landscape, and the opportunities it promises to drive are clearly recognized. As the technology continues to evolve within organizations, leaders are grappling with how best to get started in their companies’ AI adoption and acceleration. The questions we hear the most are:
- Do I need an AI leader? Should the AI leader report directly to the CEO or another C-suite leader?
- If another C-suite leader is the desired option, what part of the leadership team should AI be aligned to? How do I balance the growth potential from AI with the risks?
- Should the AI team be centrally accessible or more decentralized and aligned to the business units? What modifications need to be made across the organization so there are people from the right business units supporting the AI team?
To begin uncovering answers, the executive leadership team needs a clear understanding of their options for establishing a successful AI organization and the implications of each choice. Let’s explore the pros and cons of three different constructs.
1. The AI leader reports directly to the CEO versus being embedded deeper in the organization
Reporting directly to the CEO is more than a status-enhancing feature for the AI leader. Our research shows that some companies use this construct to underscore the importance of AI both internally and externally. This reporting line ensures the direct alignment of AI with the organization’s top-level strategies as well as the short- and long-term vision. In addition, reporting directly to the CEO elevates the visibility of AI and the AI leader’s role within the organization. This can enhance the credibility of new initiatives and elevate their importance in the eyes of internal and external stakeholders.
However, reporting directly to the CEO is not the catch-all recipe for success. Such reporting may lead to the AI leader being disconnected from the day-to-day operational challenges faced by various departments, leading to AI strategies that are not fully aligned with the practical needs of the organization. The potential for conflicts of interest among the AI leader, CEO, and business unit leaders is high, which can lead to less trust and influence on the AI leader’s part and slower implementation of new strategies, products, or services.
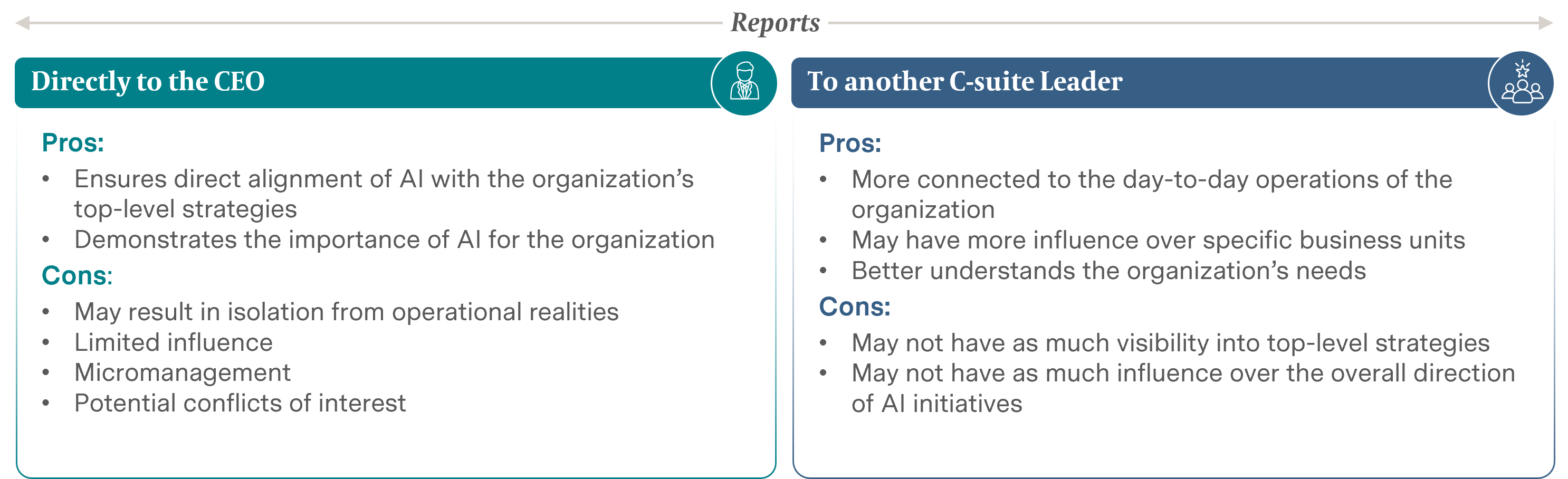
2. Alignment to cover risks versus explore opportunities
Every opportunity for business transformation involves some level of risk, and this risk becomes even more evident when integrating AI into the equation. While having the AI leader report to the CEO allows the CEO to also be close to the risks within AI’s opportunities, we have found that there are good reasons to align the AI function and AI leader role to an existing C-suite role to better balance the technical opportunities and potential risks while still demonstrating the elevated role of AI for the organization.
Across industries, we commonly see this being the Chief Technology Officer or Chief Information Officer. For many businesses, this is often a reasonable approach, as this technical leader usually has IT, technology, and innovation pipelines under their scope. Adding AI to this portfolio can produce valuable synergies around the data pipeline and IT infrastructure, the selection of high-value use cases, and the introduction of new technologies. On the other hand, the CTO/CIO organization is sometimes seen as too far detached from the business. If an AI organization is too focused on technical feasibility and not enough on commercial viability and customer desires, an AI transformation is at risk of failing.
While these technical leaders can support the organization in managing the risks associated with AI, there are also considerations for having the AI leader report to the Chief Financial Officer or Chief Risk Officer. In some highly regulated industries with large amounts of personal customer data, such as financial services or healthcare, this may be a more significant factor to weigh. Having such leaders manage AI efforts allows closer monitoring of AI-related risks while also thinking about how to use AI to effectively control risks to the organization, such as detecting insider threats.
In cases where the commerciality, the opportunities, and the forward-leading and industry-disrupting potential of AI are the top strategic priority, having reporting lines to the Chief Growth Officer or Chief Product Officer may make sense. Especially in cases where the monetization of collected data and related insights is of importance, having AI under the scope of the Chief Product Officer can be a great way to branch into new markets. In some rare cases, we have seen the Chief Operating Officer owning AI. This might happen when the core AI use cases are focused on automating processes and on internal value creation. While these leaders can provide the direction that leads AI to bring about new revenue streams, business models, or increased growth, they will need to remain focused on compliance and consider risk management.
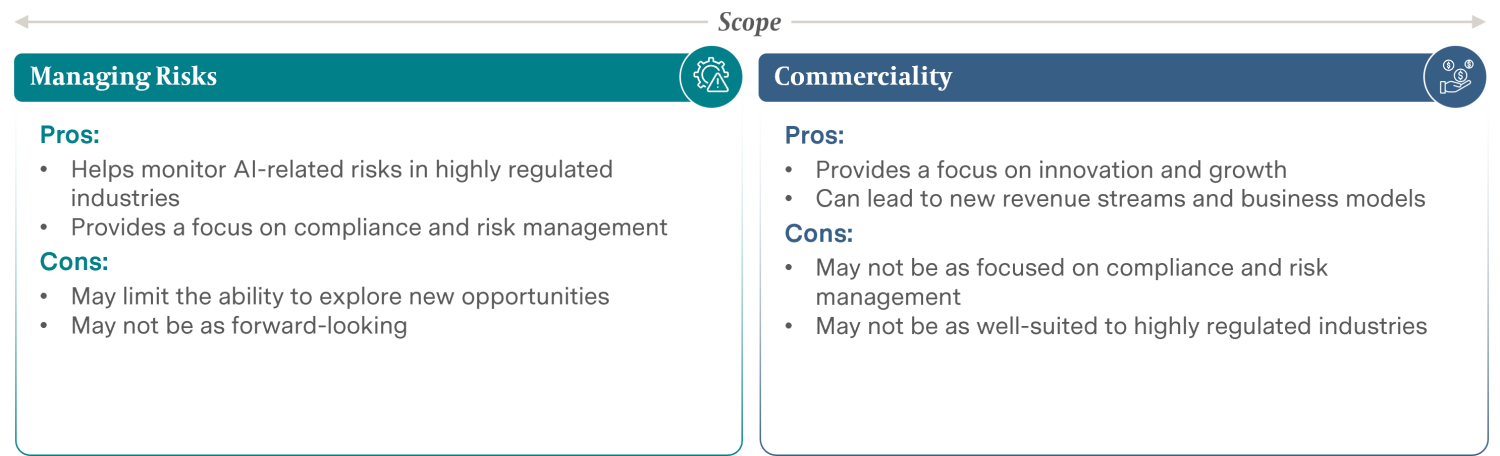
3. Optimizing the AI Organization: Centralized or Decentralized Approach
The discussion of centralized versus decentralized AI teams has been going on for years. How central the AI team is organized is a function of what makes the most sense—based not only on the company’s data but on AI maturity. It has been traditionally believed that as organizations progress with their AI maturity, they will move from centralized teams, which rapidly provide the critical mass around AI capabilities, to more decentralized teams, where the business units take over responsibility. This trend is partly true. For example, when an organization moves from experimentation in small, central teams toward implementation of use cases at scale, the business units become owners of these use cases. At that point of the AI transformation, there needs to be a sufficient number of AI professionals hired or upskilled within the business units.
We’ve found that there are occasions where this mechanism is not the driving force of an AI transformation and other factors are at play: For example, in some highly advanced industries where AI has been front and center in many parts of the business for decades, such as banking, business units likely had developed their own AI teams years ago. However, with the advent of novel and disruptive AI algorithms (e.g., large language models), some of these organizations with preexisting, highly-distributed AI teams have decided to create a small central team to focus on the possible new AI use cases for the entire company.
Other things to consider when forming an effective AI organization are that centralized teams are further away from the business. They are great at experimenting with use cases, promoting consistency in AI initiatives, and providing a point of contact for all AI-related topics. But they may be less effective when it comes to implementation and scaling because they do not consider the feasibility and the needs of individual business units. In contrast, decentralized teams are closer to the business and the customer, but efforts are often uncoordinated, which can lead to a duplication of efforts and less effective use of available investment budgets.
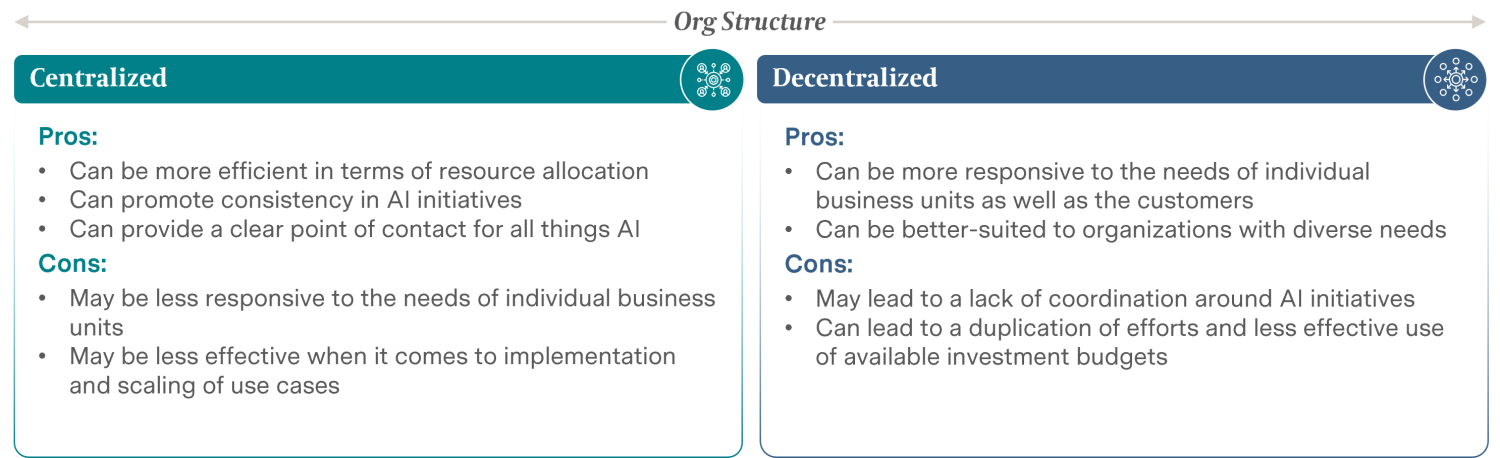
Organizations must consider how they plan to build their AI teams, bearing in mind the use of AI in internal and external initiatives, which will depend on the organization's strategic goals and objectives. Seeing that AI can improve internal processes and efficiency while also enhancing the customer experience and driving revenue growth, modifications will need to be made across the organization to ensure that technical and non-technical people from the right business units are supporting the AI leader on these initiatives. This includes establishing clear communication channels and frameworks that facilitate cross-functional collaboration. For example, the organization may consider creating a dedicated AI team or center of excellence that includes representatives from different business units, such as IT, data science, marketing, finance, legal, and customer service. This AI Center of Excellence can then work closely across business units to identify potential risks and opportunities and develop strategies to mitigate them.
The adoption and acceleration of AI within organizations require careful consideration and decision-making by executive leadership around these dichotomies. While there is no single, right answer, leaders must understand the options and implications to create effective AI roles and strategies. Finding the right balance between technical feasibility, commercial viability, and desirability is key to avoiding AI transformation failure. As AI evolves, organizations must remain flexible and adaptive to fully leverage its potential.